Research
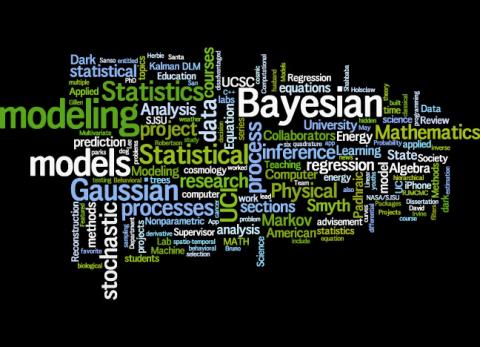
Published Papers
- A Flexible Joint Longitudinal-Survival Model for Analyzing Longitudinally Sampled Biomarkers (2021)
https://www.scirp.org/journal/paperinformation.aspx?paperid=112458
Masouleh, S.M., Holsclaw, T., Shahbaba, B., and Gillen, D.L.
Open Journal of Statistics - Bayesian Non-Homogeneous Markov Models via Polya-Gamma Data Augmentation with Applications to Rainfall Modeling (2017)
AOAS1009.pdf
- Holsclaw, T., Robertson, A., Greene, A., and Smyth. P.
Annals of Applied Statistics
(RPackage available on CRAN: \"NHMM\") - A Bayesian Hidden Markov Model of Daily Precipitation over South and East Asia (2016)
Holsclaw, T., Robertson, A., Greene, A., and Smyth. P.
Journal of Hydrometeorology - Bayesian Detection of Changepoints in Finite-State Markov Chains (2016)
Arnesen, Holsclaw, Smyth
Technometrics, Vol 58 (2), May - Measurement Error and Outcome Distributions: Methodological Issues in Regression Analyses of Behavioral Coding Data (2015)
Holsclaw, Hallgren, Steyvers, Smyth, Atkins
Psychology of Addictive Behaviors, 29(4), 1031-1040. - A Bayesian Multivariate Non-homogenous Markov Model (2014)
Greene, Holsclaw, Robertson, Smyth
Machine Learning and Data Mining Approaches to Climate Science, Proceedings of the 4th International Workshop on Climate Informatics (NCAR) - Gaussian Process Modeling of Derivative Curves (2013)
Holsclaw, Sanso, Lee, Heitmann, Habib, Higdon, Alam
Technometrics, 55(1) - Nonparametric Reconstruction of the Dark Energy Equation of State from Diverse Data Sets (2011)
Holsclaw, Alam, Sanso, Lee, Heitmann, Habib, Higdon
Physical Review D, 84, 083501, The American Physical Society. - Nonparametric Dark Energy Reconstruction from Supernova Data (2010)
Holsclaw, Alam, Sanso, Lee, Heitmann, Habib, Higdon
Physical Review Letters, 105, 241302, The American Physical Society.- Featured in: Physorg.com news article entitled "Statistical modeling could help us understand cosmic acceleration"
- Featured in: Jack Baskin School of Engineering news article entitled "Statistical analysis to explore the evolution of the universe"
- Nonparametric Reconstruction of the Dark Energy Equation of State (2010)
Holsclaw, Alam, Sanso, Lee, Heitmann, Habib, Higdon
Physical Review D, 82, 103502, The American Physical Society.
Manuscripts in Preparation
- Quantifying the Association Between Within-Subject Volatility in Serum Albumin and Mortality via a Gaussian Process Model (2017)
Holsclaw, T., Shahbaba, B., and Gillen, D.L.A - Modeling Daily Streamflow with a Periodic Auto-regressive Model with a Gaussian Process Shrinkage Prior (2017)
Holsclaw, Lall, Smyth.
UCI Team Projects
- Gaussian process modeling of longitudinal data in a survival context
Collaborators: Babak Shahbaba and Dan Gillen - UCI Statistics Department - Decadal prediction and stochastic simulation of hydroclimate over monsoonal Asia
Collaborators: Andrew Robertson (PI), Arthur Greene, and Upmanu Lall - IRI at Columbia University
Supervisor: Padhraic Smyth - UCI, Machine Learning
Research: Bayesian spatio-temporal stochastic weather generator for rainfall and temperature with multiple inputs - Automating Behavioral Coding
Collaborators: Mark Styvers (UCI) and David Atkins (UW) - Department of Psychiatry and Behavioral Science
Supervisor: Padhraic Smyth - UCI, Machine Learning
Research: stochastic modeling of time dependent discrete outcomes for behavioral data - Analysis of student behavior in Massive Open Online Courses (MOOC)
Collaborators: various - Department of Education, UCI
Supervisor: Padhraic Smyth - UCI, Machine Learning
Research: analyzing student behavior and predicting outcomes in the MOOC educational setting - Breast cancer microarray study using Lasso regression for genetic feature selection
Collaborators: Bogi Andersen - UCI School of Medicine
Supervisor: Padhraic Smyth - UCI, Machine Learning
Previous Team Projects
These some projects I worked on as a student:
- Translating Differential Equation Models Into Kernel Methods for Data Analysis - Phase III (NASA/SJSU) (Large file)
- Mathematical and Statistical Analysis of Heat Pipe Design (Intel/SJSU)
- Statistical Analysis of a Non-Linear Chemical Equation Using Numerical Methods (NASA/SJSU)
Presenstations/Posters
Dissertation - research done under the advisement of statisticians, Herbie Lee and Bruno Sanso (UCSC). The body of work covers modeling derivative curves with Gaussian processes. This work required extensive research and development of Gaussian process methods applied to inverse problems. A cosmology application drove this statistical research to model dark energy equation of state.
The dissertation was a results of an ISSDM collaboration with Los Alamos National Labs cosmologists Katrin Heitmann, Salman Habib and Ujjaini Alam and statistician David Higdon. We worked with Bayesian parametric and non-parametric models to fit non-linear equations to supernova, baryon acoustic oscilliation, and cosmic microwave backgound radiation data to elicit the form of dark energy. We have exploited the stochastic properties of the Gaussian process to perform analysis for this inverse applied problem. AOAS1009.pdf